Introduction
Over the past decade, we’ve witnessed AI technology rapidly evolve from a niche, research-oriented pursuit into a ubiquitous force reshaping industries worldwide. Today, the costs of training sophisticated AI models are plummeting—sparked by competition among cloud providers, open-source breakthroughs, and economies of scale in GPU/TPU infrastructure. Simultaneously, state-of-the-art “foundation models” can be fine-tuned or adapted with minimal overhead, drastically lowering the barrier to entry for organizations seeking AI-powered solutions.
For law firms, these trends represent an unprecedented opportunity. Far from being mere bystanders, firms can leverage their most prized asset—domain expertise in legal interpretation and strategy—to build, refine, and commercialize AI systems that tackle complex legal tasks. The confluence of commoditized AI infrastructure, cheaper model training, and advanced techniques like Reinforcement Learning from Human Feedback (RLHF) unlocks a new era of proprietary, high-value AI offerings in the legal sector.
In this blog, we explore how these trends are reshaping the business of law, highlighting the importance of RLHF, proprietary data, and expert evaluation to build best-in-class legal AI systems that can generate fresh revenue streams.
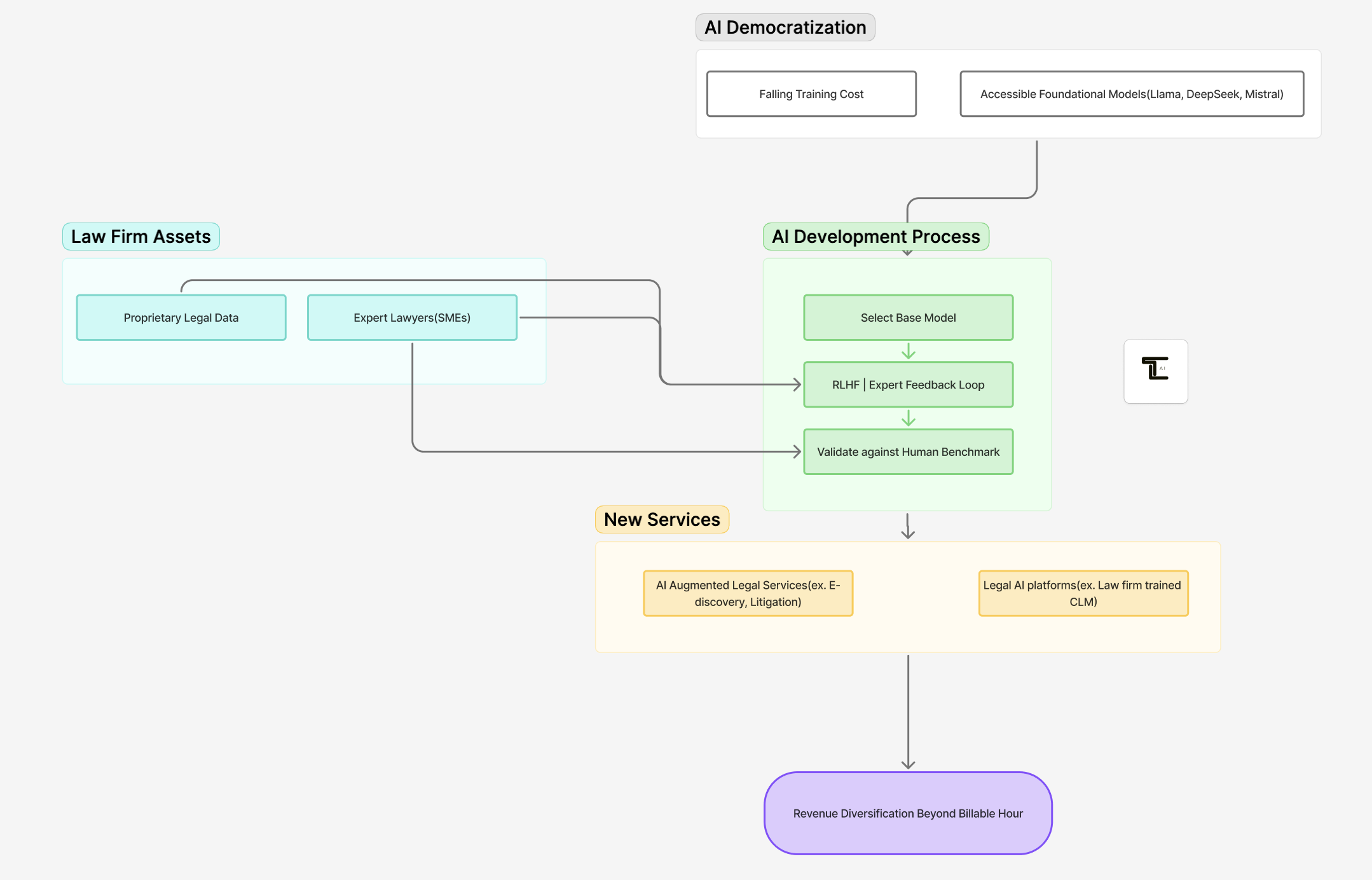
1. The Democratization (and Commodification) of AI
1.1 Lowering Costs and Simplifying Stacks
Not so long ago, training cutting-edge models was the realm of tech giants and heavily funded startups. Today, the AI infrastructure stack—compute, storage, frameworks, and tooling—has become cheaper, more accessible, and far more user-friendly. Cloud platforms compete aggressively on pricing for GPU instances, while open-source libraries (e.g., PyTorch, TensorFlow) come with robust community support and enterprise-ready solutions.
In the realm of foundation models (like GPT-based architectures or other large language models), companies can fine-tune or adapt pre-trained systems using a fraction of the resources once required. This means law firms no longer need massive R&D budgets to build sophisticated AI tools; they can start with a robust model and layer on top their legal domain expertise.
1.2 Moving Beyond Generic AI
As AI infrastructure becomes a commodity, the real differentiator is domain-specific knowledge. Generic chatbots and text completion tools are becoming widely available. But clients with complex legal challenges want accuracy, reliability, and nuance. That’s precisely where law firms can stand out—combining off-the-shelf AI components with their proprietary legal data and specialized expertise.
2. Why Law Firms Are Poised to Capitalize
2.1 Leveraging Proprietary Data
Law firms manage vast repositories of contracts, case briefs, litigation strategies, due diligence documents, memos, and more. Properly anonymized and organized, these documents form an exceptional training corpus for legal AI models. Generic large language models can provide a foundation; the firm’s unique data adds a layer of domain fidelity rarely achievable by generic AI alone.
2.2 Unmatched Subject-Matter Expertise
AI systems are only as good as the feedback they receive during training and refinement. Lawyers—particularly those with deep specialization in niches like antitrust, M&A, intellectual property, or tax law—bring contextual intelligence that even the most advanced generic AI lacks. When attorneys guide the model’s learning process, they infuse it with the subtlety and precision that high-value legal work demands.
2.3 New Revenue Opportunities
When an AI system can quickly review documents, generate tailored insights, and assist with compliance or risk assessments, it becomes an attractive offering for both existing clients and new markets. Law firms can package and commercialize these tools via:
- Subscription Models: Scalable monthly or annual fees for ongoing AI-driven support (e.g., contract analysis or compliance dashboards).
- Platform Licensing: White-label solutions licensed to in-house legal teams or smaller firms.
- Data & Analytics Services: Predictive analytics for litigation outcomes, settlement negotiation strategies, or regulatory forecasting.
These models shift the firm’s business away from the purely linear, hours-based revenue and offer higher-margin, repeatable income streams.
3. The Critical Role of RLHF (Reinforcement Learning from Human Feedback)
3.1 Why RLHF Matters in Law
Traditional model fine-tuning adjusts weights based on labeled datasets (e.g., “contract clauses labeled as risk/no-risk”), but legal work often involves complex, context-sensitive decisions. Reinforcement Learning from Human Feedback (RLHF) expands the model’s capabilities by incorporating iterative feedback from expert attorneys who interact with the model’s outputs, validating correctness, and highlighting errors in real time.
For instance:
- The AI flags a contract clause as “high risk.”
- A senior associate reviews and determines that, under specific state regulations, the clause is not high risk.
- That real-time correction feeds back into the RLHF loop, teaching the model to refine its interpretation for future similar scenarios.
3.2 Lawyer Augmentation vs. Replacement
RLHF ensures that technology remains augmented by human judgment rather than supplanting it. In highly regulated, high-stakes contexts—like corporate law, securities law, or major litigation—human oversight is essential. RLHF helps ensure the AI’s recommendations meet ethical, professional, and regulatory standards.
4. Designing Best-in-Class Legal AI Systems
4.1 Start with a Solid Foundation Model
- Select the Right Base Model: Begin with a large language model known for robust natural language understanding and generation.
- Set Clear Objectives: Determine whether your focus is on contract analysis, litigation strategy, compliance, or a broader suite of legal tasks.
4.2 Curate and Preprocess Proprietary Data
- Anonymize & Structure: Redact sensitive identifiers and organize documents by jurisdiction, practice area, or matter type.
- Metadata Tagging: Incorporate tags for complexity level, relevant regulations, risk profiles, etc., so the AI understands context more deeply.
4.3 Implement RLHF Loops
- Attorney Feedback: In-house lawyers and paralegals review AI outputs, providing granular feedback on accuracy, tone, and relevance.
- Iterative Refinement: The model continually retrains on this feedback, improving its predictions and recommendations.
- Ongoing Monitoring: Regular “check-ins” with the AI to ensure updated regulations, new precedents, and emerging best practices are integrated.
4.4 Validate, Validate, Validate
- Test Against Real-World Use Cases: Pilot solutions with select clients or internal matters, measuring time saved, accuracy, and user satisfaction.
- Comparison with Human Benchmark: Compare AI-driven reviews or analyses against senior associates or partners for a quality check.
5. Future Vision: Reinventing Law Firm Business Models
With training costs dropping and infrastructure becoming a commodity, law firms can seize the moment to evolve:
- Consulting-Plus-Tools: Offer both advanced AI tools and traditional advisory services in a single package, delivering unparalleled efficiency for clients.
- Platform Partnerships: Partner with technology vendors or cloud providers to scale proprietary AI models across global legal markets.
- Data-Driven Pricing: Embrace flat fees or subscription-based models tied to AI’s speed and accuracy, aligning costs with tangible outcomes for clients.
Ultimately, the unique competitive advantage for law firms will be their ability to blend specialized legal insight with best-in-class AI. The commodification of infrastructure and foundation models simply clears the path; it’s up to innovative firms to own the last mile of refinement, data curation, and ethical oversight.
Conclusion
As AI infrastructure and model training costs plummet, commodification is transforming how organizations adopt and scale AI. For law firms, these shifts are anything but a threat; they are a catalyst for reinvention. By harnessing proprietary data, implementing Reinforcement Learning from Human Feedback, and tapping their lawyers’ deep expertise, firms can develop truly differentiated AI solutions that create new revenue streams and client value.
Instead of being constrained by the billable hour, the law firm of the future can offer a hybrid model—part high-level advisory, part data-driven software solutions—redefining what it means to deliver legal services in the 21st century. The technology is ready, the costs are falling, and the expertise is waiting. The only question is: which firms will have the vision and the courage to act?
Interested in exploring AI-driven innovation for your law firm? Reach out for a consultation on how proprietary data, RLHF, and domain-driven refinement can power the next generation of legal services.
TABLE OF CONTENT